Debunking Common Myths About AI Training
Understanding AI Training
Artificial Intelligence (AI) is a rapidly evolving field, yet many misconceptions persist about how AI models are trained. These myths can lead to confusion and misunderstanding about the capabilities and limitations of AI. In this post, we'll explore some common myths about AI training and provide clarity on what really happens behind the scenes.
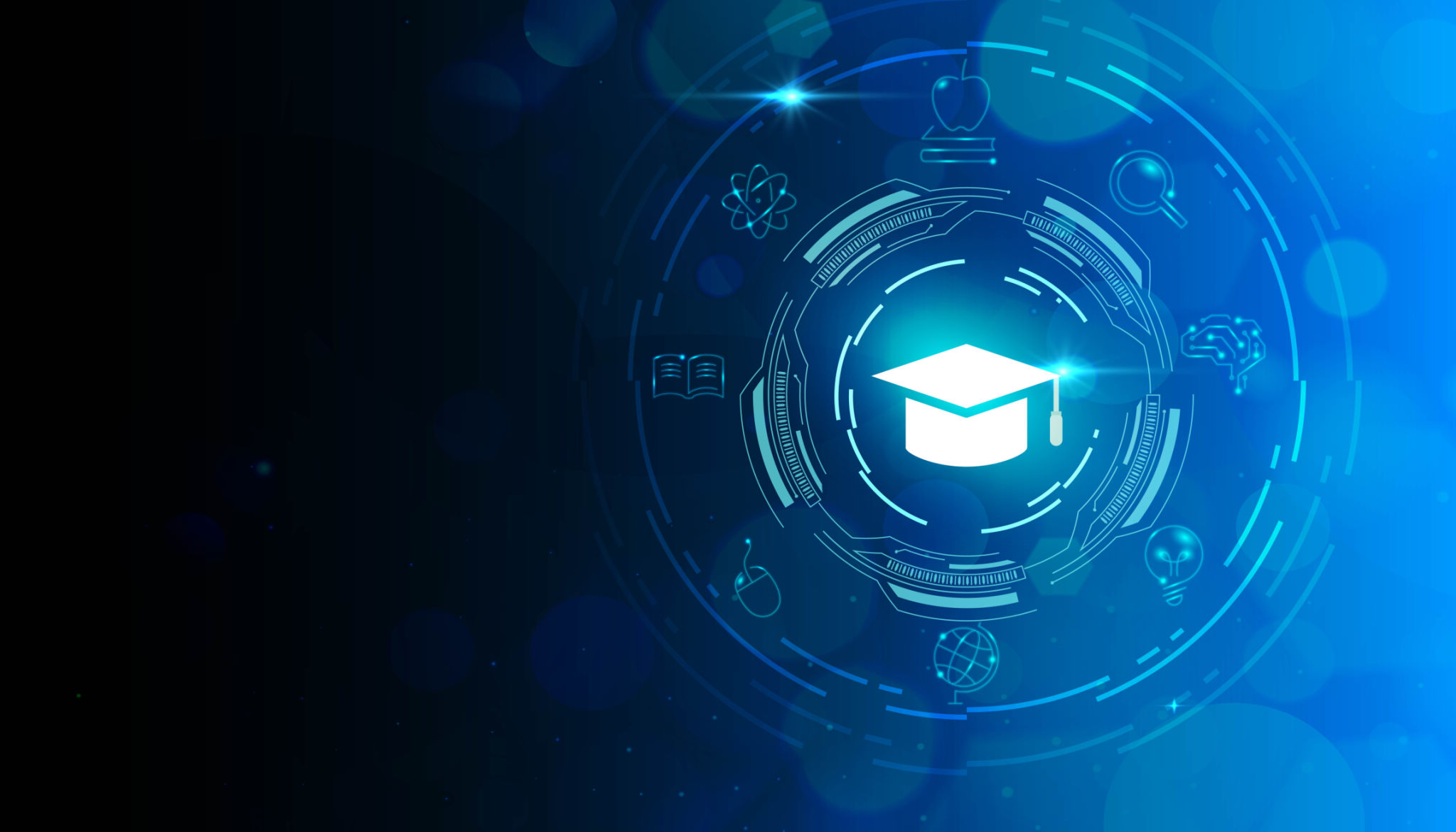
Myth 1: AI Models Train Themselves
A common misconception is that AI models can automatically train themselves without any human intervention. While AI systems can learn from data, they require significant input from data scientists and engineers to guide the training process. Experts must carefully select and preprocess data, define model architectures, and fine-tune algorithms to ensure optimal performance.
Moreover, the training of AI models involves continuous monitoring and adjustments. Human oversight is crucial to manage biases, ensure ethical considerations, and refine the models based on real-world scenarios.
Myth 2: More Data Equals Better Performance
It's often believed that feeding more data into an AI model will automatically improve its performance. While data is essential for training, quantity doesn't always translate to quality. The relevance and diversity of the dataset are far more important. An AI model trained on a diverse set of high-quality data will typically perform better than one trained on a larger but less relevant dataset.
Additionally, excessive data can lead to overfitting, where the model becomes too tailored to the training data and performs poorly on new, unseen data. Therefore, striking the right balance in data quantity and quality is key.

Myth 3: AI Training is a One-Time Process
Some believe that once an AI model is trained, the process is complete. However, AI training is an ongoing cycle. Models must be continuously updated and retrained with new data to maintain their accuracy and relevance. This iterative process ensures that AI systems can adapt to changing environments and evolving datasets.
Regular updates also help in addressing any biases or errors that may have been introduced during initial training. Continuous learning is a cornerstone of maintaining robust AI applications.
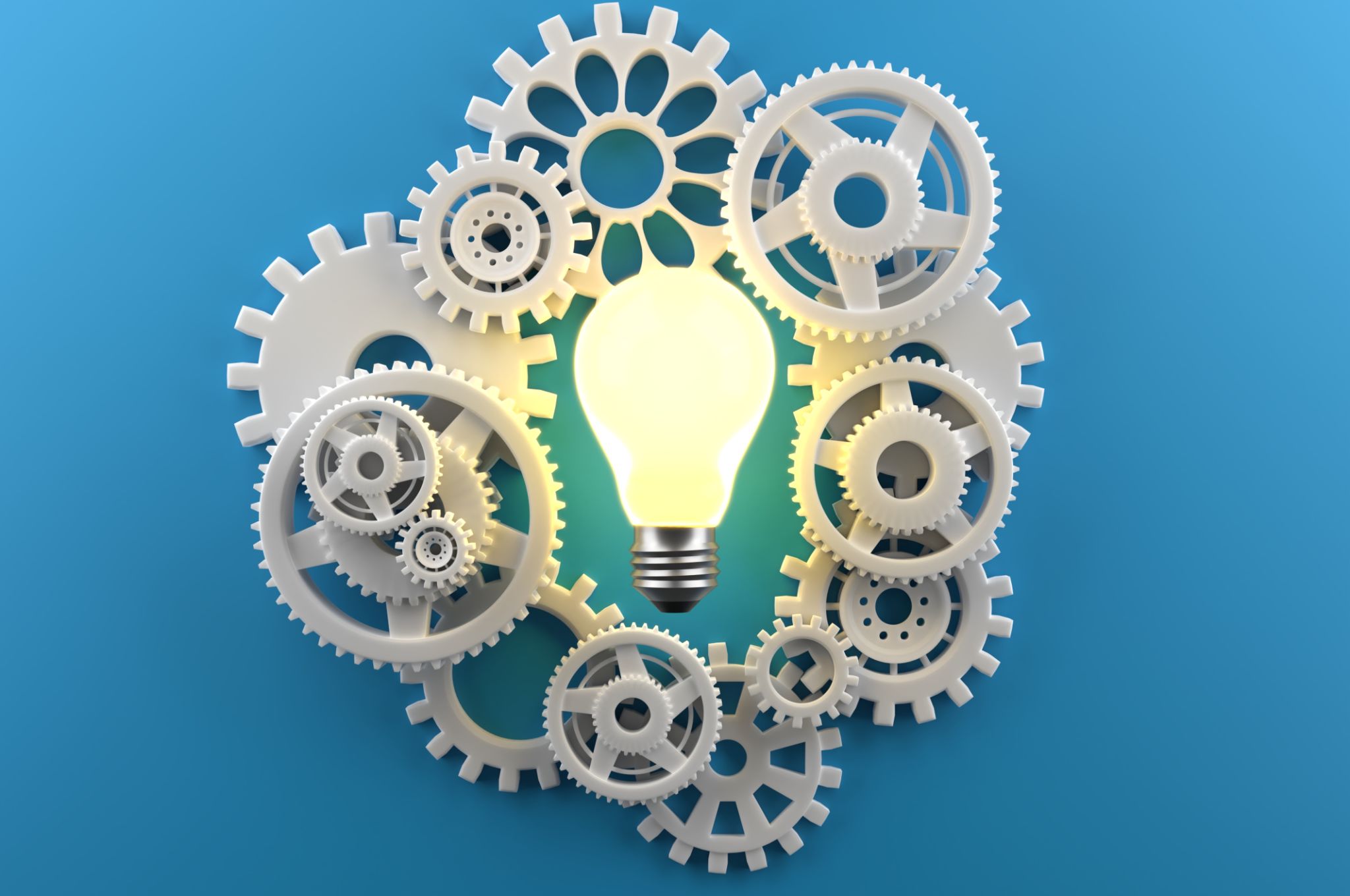
Myth 4: AI Can Learn Without Human-Labeled Data
While there are techniques like unsupervised learning that don't rely on labeled data, many powerful AI models still require large amounts of human-labeled data for training. Labeled datasets provide the necessary context for models to understand complex patterns and make accurate predictions.
Human involvement in labeling ensures that the data reflects real-world conditions and nuances, which are essential for developing effective AI systems.
Myth 5: AI Training is Just About Algorithms
The focus on algorithms often overshadows other critical aspects of AI training, such as infrastructure and computational resources. Training complex models requires substantial computing power, often involving specialized hardware like GPUs or TPUs to handle the computations efficiently.
Furthermore, deploying and maintaining AI models involves considerations such as scalability, security, and integration with existing systems. Thus, successful AI training encompasses a holistic approach beyond just algorithms.
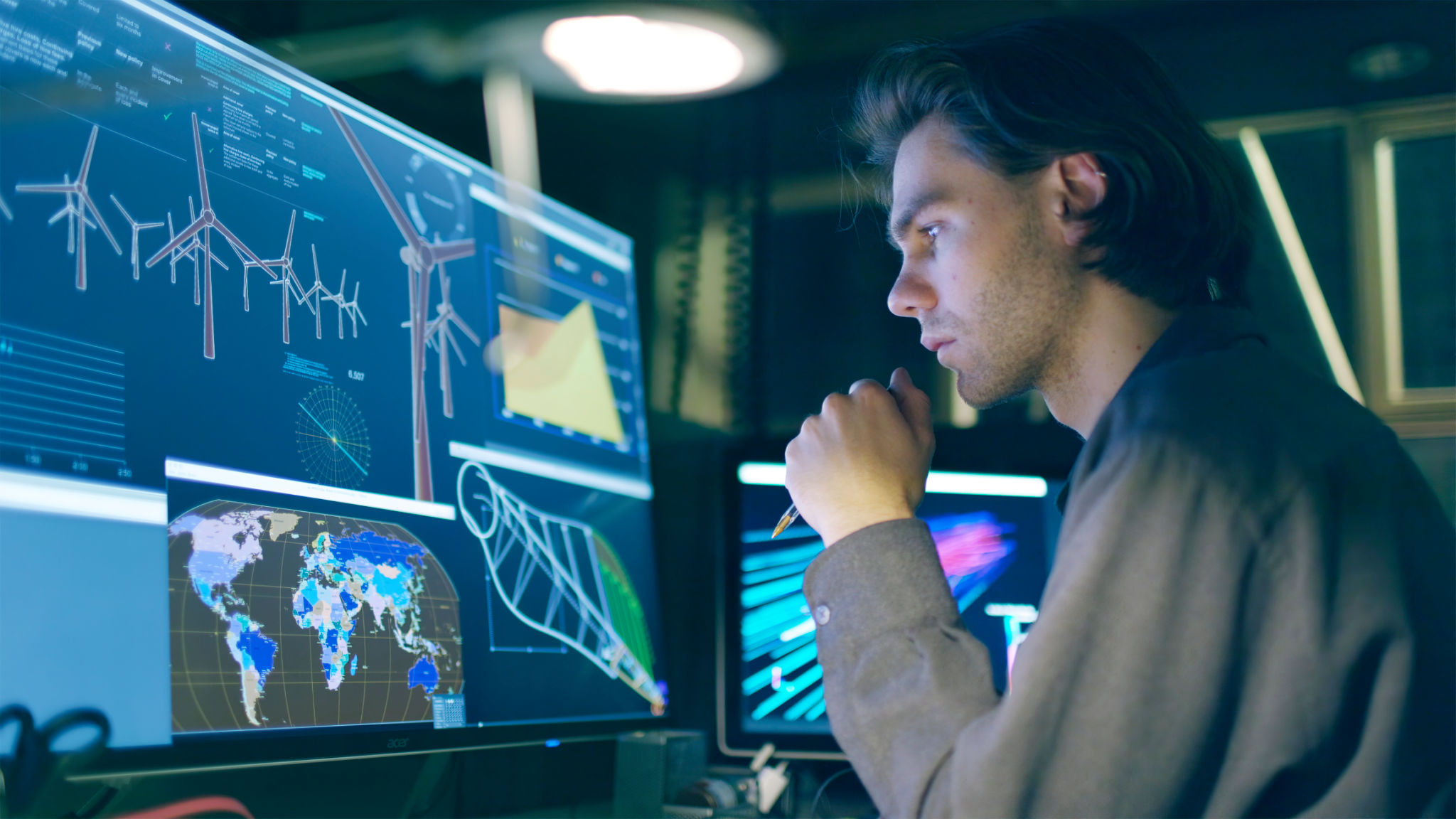
Conclusion
Debunking these myths is essential for a realistic understanding of AI training. Recognizing the multifaceted nature of AI development helps stakeholders make informed decisions about implementing and utilizing AI technologies effectively. As we continue to advance in the field of artificial intelligence, clarity about its processes will be crucial for harnessing its full potential.